
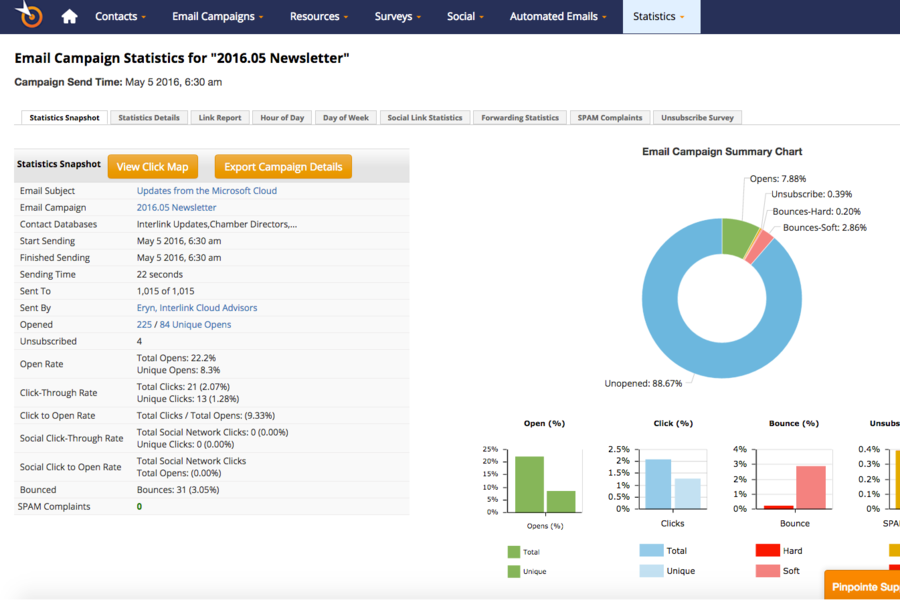
It includes errors in questionnaire design like question order bias, where the wording or theme of previous questions affect how a respondent answers later in the survey. Non-sampling error is where there’s a problem with how the survey is set up and carried out. It just means there will be a margin of error in your results. Some degree of sampling error is inevitable, but it doesn’t mean survey research is not worth doing. It’s the extent to which the sample differs from the population. Sampling error is a natural effect of using a sample to study a larger population. We can sort survey error into two types – sampling and non-sampling error. If these confidence intervals grow too large, the quality of the data and inferences can be degraded to the point of making them uninformative – and meaning your time and energy conducting a survey is wasted. Increased error typically results in larger confidence intervals (reduced certainty) around the estimates in the data and inferences made about the population of interest. As a result, understanding survey errors is key to understanding survey data quality. Survey errors reduce, but don’t necessarily eliminate, our ability to accurately make inferences about the larger population from our sample. Evaluations of survey data quality typically reflect how successful the project has been in doing that. The goal of a survey is usually to make inferences about a larger population of interest – using a sample to research a population. As well as the human mistakes in surveying, when we talk about error we’re talking about degrees of accuracy and certainty in our data.Įrrors are sources of uncertainty, both in the estimates in the data and the conclusions we draw from that data. But when it comes to data, the definition is a little broader. In everyday language, error just means a mistake.
